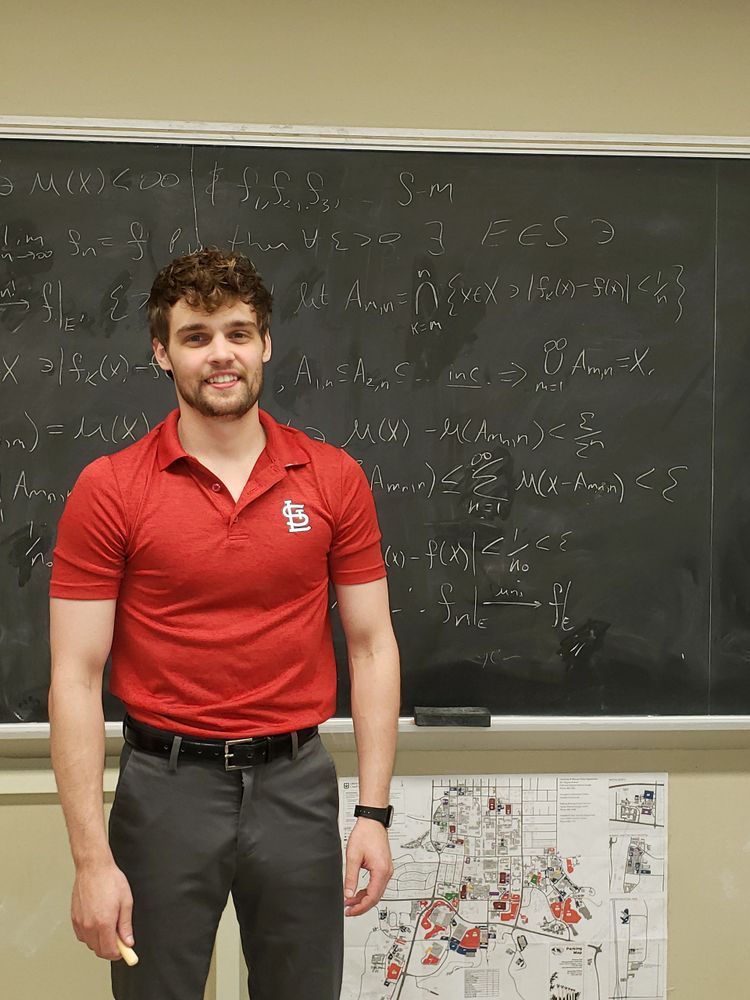
My research interests center around Harmonic Analysis with topics such as singular integral operators, multilinear estimates, Littlewood-Paley theory, weighted norm inequalities, almost everywhere convergence, and broader function space estimates.
In this paper, we establish a normed fractional Faà di Bruno inequality within the framework of Lebesgue spaces. This extends the classical fractional chain rule from the range 0 < s < 1 to an arbitrarily large value of s. Download
In this paper we establish a fractional chain rule in the setting of weighted Triebel-Lizorkin spaces under various smoothness conditions. Notably, this provides a fractional chain rule for weighted Lebesgue spaces, L^p(w), for p\leq 1. Additionally, an explicit relationship between the smoothness index, integrablity index, and the choice of weights is determined. Download
We prove almost everywhere convergence for convolutions of locally integrable functions with shrinking L^1 dilations of a fixed integrable kernel with an integrable radially decreasing majorant. The set on which the convergence holds is an explicit subset of the Lebesgue set of the locally integrable function of full measure. This result can be viewed as an extension of the Lebesgue differentiation theorem in which the characteristic function of the unit ball is replaced by a more general kernel. We obtain a similar result for multilinear convolutions. Download
We prove the Kato-Ponce inequality (normed Leibniz rule) for multiple factors in the setting of multiple weights (A_{\vec P} weights). This improves existing results by extending the class of known weights for which the inequality holds. Download
In this paper, we establish weighted Kato-Ponce inequalities for multiple factors. Our work is carried out in the framework of Muckenhoupt weights with particular focus on the weighted L^1 endpoint case. In this work we also extend the validity of the Kato-Ponce inequality from the class of Schwartz functions to the broader class of weighted Sobolev spaces. Download
We extend the Kato-Ponce inequality to a product of m functions, proving an estimate currently missing from the literature. This study is motivated by the fact that the 3-factor Kato-Ponce does not follow directly from the 2-factor version in the full range of permissible indices. Our methodology is based upon that by Grafakos and Oh but our extension entails a novel decomposition that elegantly and effectively handles the technical difficulties that arise from the combinatorial complexity of the possibly large number of factors. Download
We use cookies to analyze website traffic and optimize your website experience. By accepting our use of cookies, your data will be aggregated with all other user data.